
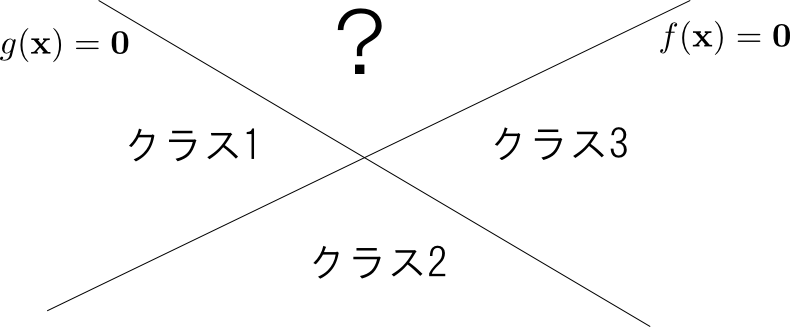
Galar, M., Fernandez, A., Tartas, E.B., Sola, B., Herrera, F.: Dynamic classifier selection for one-vs-one strategy: avoiding non-competent classifiers. 17(3), 696–704 (2006)įriedman, J.H.: Another approach to polychotomous classification. USA 92, 8700–8704 (1995)įei, B., Liu, J.: Binary tree of SVM: a new fast multiclass training and classification algorithm. 2, 263–286 (1995)ĭubchak, I., Muchnik, I., Holbrook, S.R., Kim, S.H.: Prediction of protein folding class using global description of amino acid sequence.
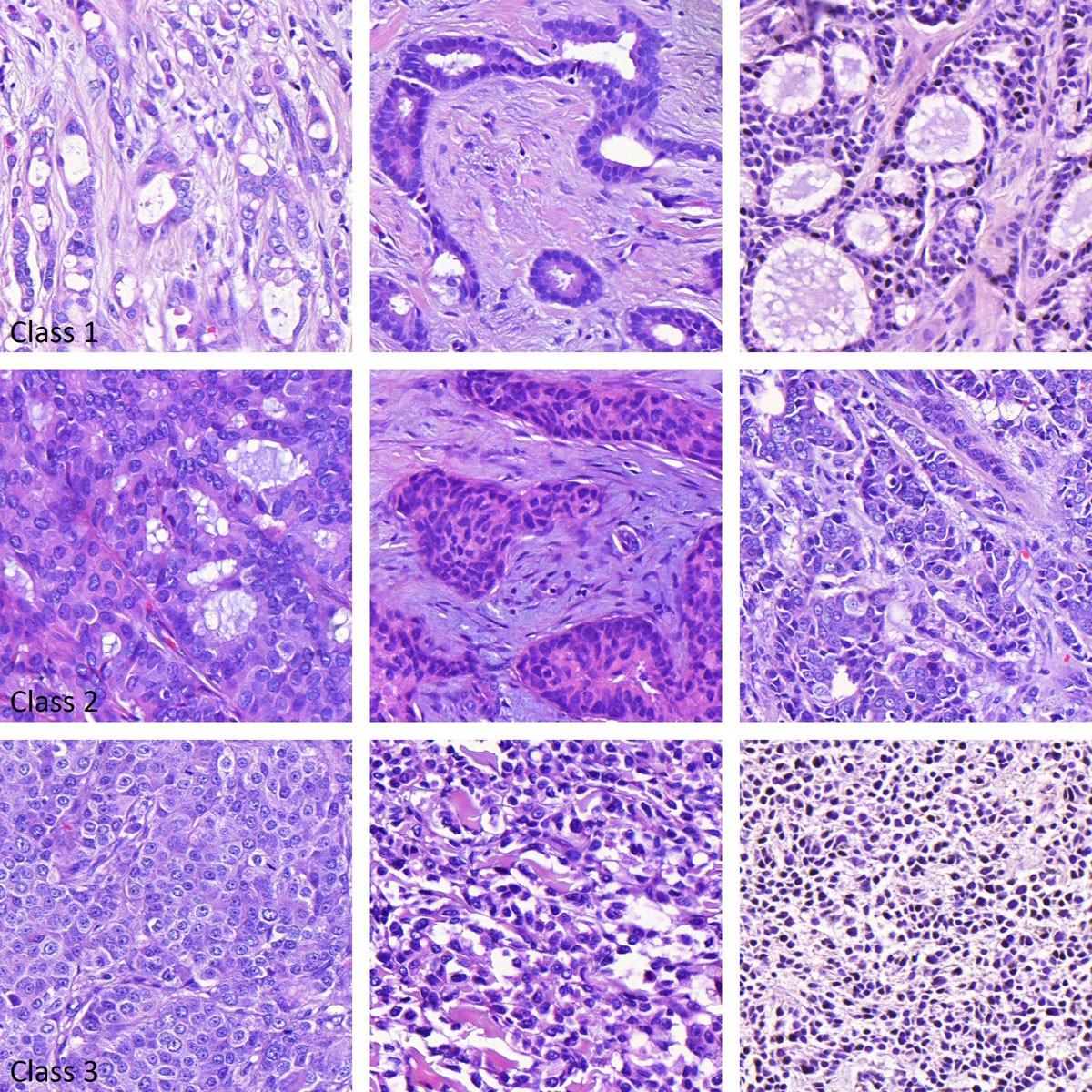
Bioinformatics 17, 349–358 (2001)ĭietterich, T.G., Bakiri, G.: Solving multiclass problems via error-correcting output codes. 20(2), 200–211 (2012)ĭing, C.H., Dubchak, I.: Multi-class protein fold recognition using support vector machines and neural networks. In: Proceedings of XLIII Conference on Mathematics Applications, in Polish (2014)Ĭhmielnicki, W., Roterman-Konieczna, I., Stapor, K.: An improved protein fold recognition with support vector machines. Neurocomputing 75(1), 194–198 (2012)Ĭhmielnicki, W., Stapor, K.: A modification of the pairwise coupling algorithm to solve multi-class problem. 6076, 162–169 (2010)Ĭhmielnicki, W., Stapor, K.: A hybrid discriminative/generative approach to protein fold recognition. 5, 1143–1175 (2004)Ĭhmielnicki, W., Stapor, K.: Protein fold recognition with combined SVM-RDA classifier.
#One versus rest synonym software
Software available at (2001)Ĭhen, D.R., Wu, Q., Ying, Y., Zhou, D.X.: Support vector machine soft margin classifiers: error analysis. 1, 113–141 (2001)Ĭhang, C.C., Lin, C.J.: LIBSVM: a library for support vector machines. This process is experimental and the keywords may be updated as the learning algorithm improves.Īllwein, E., Schapire, R., Singer, Y.: Reducing multiclass to binary: a unifying approach for margin classifiers. These keywords were added by machine and not by the authors. Moreover, the proposed method turns out to be much more efficient than the original one-versus-one strategy. The results show that we obtain better recognition ratio on all tested databases. We used SVM classifier on several different databases to test our solution. As the performance we understand both recognition ratio and the speed of the proposed algorithm. In this paper, we try to combine theses strategies to exploit their strong aspects to achieve better performance. There are several papers dealing with these methods, improving and comparing them. Two of the widely known are one-versus-one and one-versus-rest strategies. There are many methods of decomposition such a task into the set of smaller classification problems involving two classes only. Support Vector Machine (SVM) is a binary classifier, but most of the problems we find in the real-life applications are multiclass.
